Publications
Space-Time Models based on Random Fields with Local Interactions
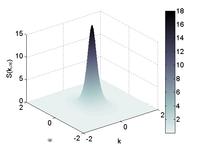
The analysis of space-time data from complex, real-life phenomena requires the use of flexible and physically motivated covariance functions. In most cases, it is not possible to explicitly solve the equations of motion for the elds or the respective covariance functions. In the statistical literature, covariance functions are often based on mathematical constructions. We propose deriving space-time covariance functions by solving effective equations of motion", which can be used as statistical representations of systems with diffusive behavior. In particular, we propose using the linear response theory to formulate space-time covariance functions based on an equilibrium effective Hamiltonian. The effective space-time dynamics are then generated by a stochastic perturbation around the equilibrium point of the classical field Hamiltonian leading to an associated Langevin equation. We employ a Hamiltonian which extends the classical Gaussian field theory by including a curvature term and leads to a diffusive Langevin equation. Finally, we derive new forms of space-time covariance functions.
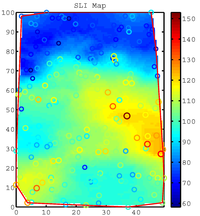
Stochastic Local Interaction (SLI) Model: Interfacing Machine Learning and Geostatistics
D. T. Hristopulos,
Abstract: Machine learning and geostatistics are two powerful approaches used to model spatial data. Each approach has advantages and disadvantages, but they both suffer from poor scaling of the computational load with data size due to the inversion of large covariance matrices. We present a new method for the modeling of spatial data that combines ideas from statistical physics, computational geometry, and machine learning. The proposed Stochastic Local Interaction (SLI) model is based on an explicit precision (inverse covariance) matrix and can be applied to data in n-dimensional spaces. The SLI model is defined by means of a Gaussian joint probability density function, which is expressed in terms of energy functionals that involve local interaction constraints implemented by means of kernel functions. The variability of the sampling density is accounted by means of local kernel bandwidths. The SLI model leads to a semi-analytical expression for interpolation (prediction), which is valid in any number of dimensions and does not require the inversion of a covariance matrix.
Radial Covariance Functions Motivated by Spatial Random Field Models with Local Interactions
D. T. Hristopulos, Stoch Environ Res Risk Assess (2015) 29:739–754
Abstract: We derive explicit expressions for a family of radially symmetric, non-differentiable, Spartan covariance functions in two dimensions that involve the modified Bessel function of the second kind. In addition to the characteristic length and the amplitude coefficient, the Spartan covariance parameters include the rigidity coefficient η1 which determines the shape of the covariance function. If η1>>1 Spartan covariance functions exhibit multiscaling. We also derive a family of radially symmetric, infinitely differentiable Bessel-Lommel covariance functions valid in any spatial dimension greater than or equal to two. We investigate the parametric dependence of the integral range for Spartan and Bessel-Lommel covariance functions using explicit relations and numerical simulations. Finally, we define a generalized spectrum of correlation scales in terms of the fractional Laplacian of the covariance function. This spectrum extends from the smoothness microscale to the integral range. The smoothness scale of mean-square continuous but non-differentiable random fields vanishes; such fields, however, can be discriminated by means of the generalized correlation scale.
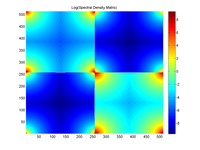
Multivariate Spartan Spatial Random Field Models
D. T. Hristopoulos and E. Porcu, Probabilistic Engineering Mechanics, Volume 37, July 2014, pp. 84–92
Abstract: This paper introduces a family of stationary multivariate spatial random fields with D scalar components that extend the scalar model of Gibbs random fields with local interactions (i.e., Spartan spatial random fields). We derive permissibility conditions for Spartan multivariate spatial random fields with a specific structure of local interactions. We also present explicit expressions for the respective matrix covariance functions obtained at the limit of infinite spectral cutoff in one, two and three spatial dimensions. Finally, we illustrate the proposed covariance models by means of simulated bivariate time series and two-dimensional random fields.